Federated Learning for Edge AI: Realistic Hardware-Software System Co-Design
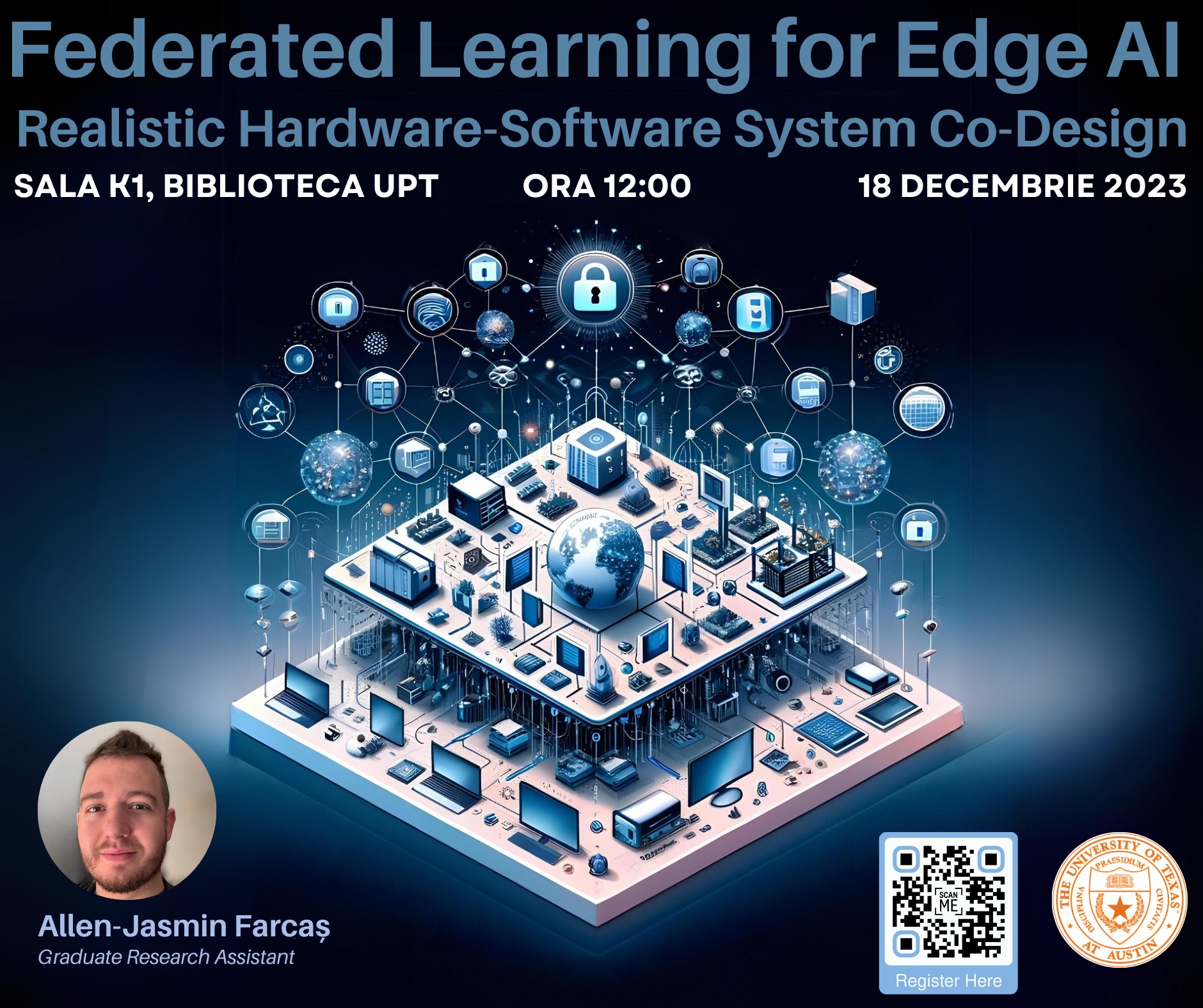
Federated Learning (FL) is essential in the rapidly expanding Internet-of-Things (IoT) landscape. The addition of nearly 2 billion devices in 2023 has intensified the need for Edge AI to adopt efficient FL solutions, thus enabling decentralized ML model training on edge devices with a focus on data privacy.
My research focuses on Hierarchical Federated Learning (HFL), a variant of FL that leverages edge servers that are physically closer to end-user devices. HFL is better fit for real-world scenarios since it reduces communication costs and is more scalable.This talk will explore the synergies between hardware-software co-design and real world considerations such as user mobility and semi-supervised learning solutions in and FL, showing how these innovations can lead to more efficient, scalable, and privacy-centric IoT ecosystems.
Biography: Allen-Jasmin Farcaș is a fourth-year Ph.D. student at The University of Texas at Austin. His research interests include federated learning systems, edge AI systems, and hardware/software co-design for edge AI. Farcas received his bachelor’s degree in computer engineering in 2019 from Politehnica University Timisoara.
When & Where: Wed, December 18th, Biblioteca UPT, K1 amphitheater.
Attendance is free, please register here: https://forms.gle/F7GVDkpGjVHAxyVt9
Organizing team,
IEEE SSIT